Support tools to guide decision making in precision medicine are getting more attention, especially as payers and providers have become more aware of the challenges with reporting and interpretation of genetic analyses
In another example of building infrastructure for precision medicine programs, researchers from the Children’s Hospital of Philadelphia (CHOP) have developed a new tool to augment human interpretation of precision medicine data. This tool is designed to help oncologists and other clinicians distinguish clinically important genetic data from a large quantity of data that is abnormal, but not clinically relevant.
A serious problem clinicians face is interpreting and reporting the vast amount of data generated by next-generation sequencing (NGS). While testing can identify millions of different genomic variants, determining which variants have clinical meaning for facilitating precision medicine has become impractical and time consuming.
To solve this problem and make precision medicine easier to adopt into clinical practice, CHOP researchers have developed CancerVar. CancerVar uses machine learning technology to not only identify cancer mutations, but to also interpret the significance of the mutations that the tool identifies.
Machine Learning Tool Produces 3 Critical Distinctions for Somatic Variants
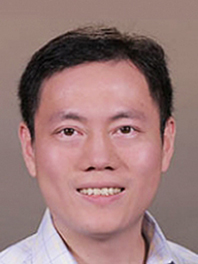
Genomic information-based disease prognosis and specific genotypic and biological biomarkers, combined with other therapeutic strategies based on the tumor biology, have been studied at CHOP for several years. Recent progress has been outlined in a study published in Science Advances, the authors explaining that the new CancerVar platform distinguishes cancer mutations in terms of three critical distinctions: cancer diagnosis, prognosis, and targetability.
According to the authors, CancerVar involves a user-friendly web server with precomputed clinical evidence for 13 million variants from 1,911 cancer census genes through literature mining and database aggregations.
With this and their previous work, the CHOP researchers have sought to standardize and improve interpretations for somatic mutations based on the Association of Molecular Pathology (AMP), American Society of Clinical Oncology (ASCO), and the College of American Pathologists (CAP) rule-based score system, and other options, according to the article.
Reduces the Labor Burdens for Pathologists
“CancerVar will not replace human interpretation in a clinical setting, but it will significantly reduce the manual work of human reviewers in classifying variants identified through sequencing and drafting clinical reports in the practice of precision oncology,” said Kai Wang, PhD, in a CHOP statement. Wang is a Professor of Pathology and Laboratory Medicine at CHOP and senior author of the Science Advances paper.
“Somatic variant classification and interpretation are the most time-consuming steps of tumor genomic profiling,” added Marilyn M. Li, MD, MS, co-author of the study. “CancerVar provides a powerful tool that automates these two critical steps. Clinical implementation of this tool will significantly improve test turnaround time and performance consistency, making the tests more impactful and affordable to all pediatric cancer patients.” Li is a Professor of Pathology and Laboratory Medicine and Director of Cancer Genomic Diagnostics at CHOP.
While the authors acknowledged limitations with CancerVar, the goal is to help clinicians quickly prioritize treatments based on precision medicine data. The machine learning tool documents and harmonizes various types of clinical evidence including drug information, publications, and pathways for somatic mutations in detail, according to Wang. “By providing standardized, reproducible, and precise output for interpreting somatic variants, CancerVar can help researchers and clinicians prioritize mutations of concern.”
Another New Tool Takes Condition-Specific Approach
The developers of another precision medicine workflow tool explain that it targets complex, condition-specific genetic mutations, in this case, inflammatory bowel disease (IBD). The work marks a potential turning point for researchers and clinicians from the United Kingdom-based Quadram Institute, the Earlham Institute, the Norfolk and Norwich University Hospital and University of East Anglia, and collaborators in Cambridge, London, and Leuven (Belgium).
Using single nucleotide polymorphisms (SNP), or genomic variants, in an integrated SNP Network Pipeline (iSNP), the artificial intelligence (AI) program appears to identify hidden connections between the genetic drivers of SNPs in inflammatory bowel disease. The paper outlining iSNP and how it supports clinicians was published in Nature Communications.
“Until now there were only a few tools that could carry out a complex analysis on a patient-specific way and provide various molecular maps of the same disease,” said researcher leader Simon Carding, PhD, in a Quadram Institute statement. “With iSNP, it is now a reality to screen large cohorts, understand better the individual pathogenesis stories, and find the best treatment for the right patient.”
As Publication of Curated Sequencing Data Continues
Both of these examples highlight how decision-making tools are developing from curated genomic data. Whether they are to improve knowledge of medical utility or to accelerate drug target discovery, the increasing number of genomic databases are not without some cautions.
In “Standards and Guidelines for the Interpretation and Reporting of Sequence Variants in Cancer,” Li and others offered several steps for clinical laboratories, including:
• Understanding the content of the database and how the data are aggregated, including reviewing documentation and published literature;
• Paying specific attention to the limitation of each database and the methods used; and
• Assessing the quality of the provided genomic data, based on the source, in combination with verifying data quality of the pathological diagnosis provided (e.g. site, diagnosis, and subtype).
By staying aware of and assessing the quality of new interpretation and reporting tools for decision making in precision medicine, healthcare leaders will be best positioned to develop programs that serve patients well and meet payer expectations for reimbursement. Ultimately, tools like CancerVar and iSNP will support clinicians wanting to personalize care and tailor treatments for individual patients.
—Caleb Williams
Related Information:
Uncovering Hidden Genetic Connections for Personalised Medicine
Standards and Guidelines for the Interpretation and Reporting of Sequence Variants in Cancer