Hospitals performing precision medicine research using a machine learning algorithm or AI-based personalized medicine techniques may be able to apply Georgia Tech’s findings in a way that improves their current machine learning methods
In another example of building predictive tools for personalized medicine, the Georgia Institute of Technology (Georgia Tech) and the Ovarian Cancer Institute have developed a new machine learning (ML) algorithm strategy to predict how ovarian cancers will respond to different drugs. The point made in this precision medicine study is that ML algorithms are more powerful when multiple different algorithms are used.
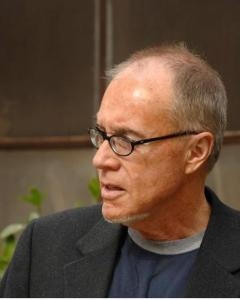
“In medicine, we need to be able to make predictions,” said John F. McDonald, PhD, one of the study’s leaders, in a Georgia Tech article. McDonald is a professor in the School of Biological Sciences and director of the Integrated Cancer Research Center in the Petit Institute for Bioengineering and Bioscience at the Georgia Institute of Technology.
“In analyzing complex datasets in cancer biology, we can use machine learning, which is simply a sophisticated way to look for correlations,” McDonald said. “The advantage is that computers can look for these correlations in extremely large and complex data sets.”
Predictive Machine Learning Algorithm Used for Ovarian Cancer Precision Medicine
McDonald’s team applied machine learning to predict what medications would best treat different ovarian cancers. However, instead of using a single machine learning algorithm, the research team used multiple different algorithms to obtain ovarian cancer precision medicine data. The related article was published in the Journal of Oncology Research.
“The goal of precision cancer medicine is to optimize clinical efficacy by customizing therapies to individual patients,” the researchers wrote. “Machine-learning algorithms have emerged as useful tools to accomplish this goal, but predictive performance of single-model approaches remain inadequate.” The novel approach of combining multiple machine learning algorithms seems to have paid off.
“While additional validation will need to be carried out using larger numbers of patients with multiple types of cancer,” McDonald said, “our preliminary finding of 90% accuracy in the prediction of drug responses in ovarian cancer patients is extremely promising and gives me hope that the days of being able to accurately predict optimal cancer drug therapies for individual patients is in sight.”
Machine Learning Algorithm Uses Gene Expression Profiling to Personalize Ovarian Cancer Treatment
Points of interest from Georgia Tech’s ensemble machine learning algorithm study include:
- Five different machine learning algorithms were tested, with an ensemble classifier constructed to determine the final prediction.
- More than two million simulations were run to optimize the different components of the machine learning algorithm to develop the final ELAFT model.
- Combining gene expression and proteomics features together improved prediction accuracy marginally.
“In our approach, we utilize an ensemble of machine-learning methods to build predictive algorithms—based on correlations between gene expression profiles of cancer cell lines or patient tumors with previously observed responses—to a variety of cancer drugs,” McDonald said.
The eventual goal is to feed the algorithms with the gene expression profiles of tumor biopsies, McDonald added. The algorithms would then be expected to predict, with high accuracy, patient responses to different drug therapies. For ovarian cancer, though, the researchers noted the importance of this specific work.
Need to Better Predict Efficacy of First-Line Therapies
“Right now, a percentage of patients will not respond to a drug, but we don’t know that until after six weeks of chemotherapy,” said McDonald. “What we hope is that we will soon have tools that can accurately predict the probability of a patient responding to first line therapies—and if they don’t respond, to be able to make accurate predictions as to the next drug to be tried.”
—Caleb Williams
Related Information:
Multi-Algorithm Approach Helps Deliver Personalized Medicine for Cancer Patients