Artificial intelligence (AI)-driven advances in precision medicine are accelerating potential treatments for incurable neurological diseases
While scientists are striving to find cures for neurological diseases such as Parkinson’s disease, and amyotrophic lateral sclerosis (ALS) or Lou Gehrig’s disease, these conditions are difficult to track and manage. The good news is that artificial intelligence (AI) technologies are increasing the detection, treatment, and monitoring options available to healthcare leaders and neurologists.
Watching a New Set of Genes for ALS Treatment Connections
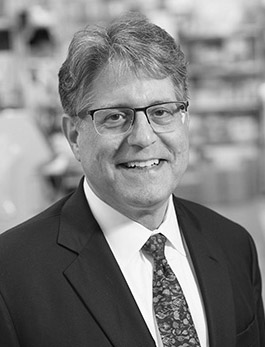
A recent study using AI found 28 new potential genetic targets for treating ALS, a progressive neurological disease that is incurable and leads to complete paralysis. Eighteen of the genetic targets identified were validated using biological models, offering researchers multiple new potential methods of treating ALS.
Recently published in Frontiers in Aging Neuroscience, the study involved an international initiative and included researchers from Johns Hopkins University School of Medicine, Massachusetts General Hospital, Harvard Medical School, Mayo Clinic, and Tsinghua University.
This study leveraged a large dataset collected by Answer ALS, one of the largest ALS research projects currently in existence. The dataset was analyzed using software called PandaOmics, an AI-enabled engine of Insilico Medicine’s Pharma.AI platform which integrates proteomics, transcriptomics, and other data types with advanced AI algorithms.
“We are truly excited to see the Answer ALS data being used to identify possible ALS disease-causing pathways and candidate drugs,” said Jeffrey D. Rothstein MD, PhD, Professor of Neurology at Johns Hopkins and Director of the Robert Packard Center for ALS Research and Answer ALS, in an Insilico press release announcing the new findings. “The work by Insilico is exactly how this unprecedented program was envisioned to help change the course of ALS.”
This research initiative used an AI-driven tool to identify targets and then validated the findings using biological models. The approach allowed an efficient method of leveraging data to quickly identify new treatment targets.
“From AI-powered target discovery based on massive datasets, to biological validation by multiple model systems (fly, mouse, human iPS cells), to rapid clinical testing through investigator-initiated trials (IIT), this represents a new trend that may dramatically reduce the costs and duration and more importantly the success rate of developing medicines, especially for neurodegenerative diseases” explained Bai Lu, PhD, Professor in the School of Pharmaceutical Sciences at Tsinghua University. “We are very happy to be part of this international team, and very excited about the subsequent efforts to clinically validate these novel targets.”
Added Alex Zhavoronkov, PhD, Founder and CEO of Insilico Medicine, “The results of this collaborative research effort show what is possible when we bring together human expertise with AI tools to discover new targets for diseases where there is a high unmet need. This is only the beginning.” Zhavoronkov indicated that the AI technology his team has developed could be applied to other fields of medicine, including oncology, immunology, and fibrosis.
Using Remote Sleep Monitoring for Detecting Parkinson’s Breathing Patterns
While AI has provided numerous new treatments for ALS, another AI tool, developed by researchers at the Department of Electrical Engineering and Computer Science (EECS) at MIT and the MIT Jameel Clinic, has resulted in a new way of detecting and monitoring Parkinson’s disease.
Published in Nature Medicine, this study used a small remote device that could detect patients’ movements and breathing patterns. AI analysis of this data found that by monitoring breathing, the device could help provide early recognition of the presence of Parkinson’s disease.
“Breathing patterns refer to the sequence of inhales and exhales, the details of the corresponding chest movements, and their progression throughout the night as the person enters different sleep stages and cycles,” said Dina Katabi, PhD, the study’s senior author, in a recent interview with Forbes. “With training, the algorithm was able to detect complex patterns that identify people who have Parkinson’s from those who don’t.”
This AI advance uses a technique that would not be humanly possible, potentially allowing the disease to be diagnosed much earlier than current techniques. “Parkinson’s disease diagnosis relies on motor symptoms like tremors and stiffness, however, those symptoms tend to appear several years after the onset of the disease,” explained Katabi. “But, no physician today can detect Parkinson’s or assess its severity merely from breathing.”
“AI can help doctors and medical professionals extract new insights from standard physiological signals like breathing, heart rhythms, electrical activity, gait or walking patterns,” continued Katabi. “This is because AI, or neural networks, can detect complex patterns that may be hard for humans to see in this kind of data.”
The mobility of this technology adds another layer of value. “By being able to have a device in the home that can monitor a patient and tell the doctor remotely about the progression of the disease, and the patient’s medication response so they can attend to the patient even if the patient can’t come to the clinic—now they have real, reliable information—that actually goes a long way toward improving equity and access,” Katabi said in an MIT press release.
Another advantage of this technology is that it enables better tracking of patients’ responses to medications. “This enables us to objectively measure how your mobility responds to your medication,” explained Yingcheng Liu, a PhD student at MIT who was co-lead author of the study. “Previously, this was very cumbersome to do because this medication effect could only be measured by having the patient keep a journal.”
Accelerating AI in Biomedical Research and Healthcare
Although there have been strides in developing artificial intelligence for healthcare in recent years, understanding how best to deploy AI for neurological diseases will take time. Among the challenges to widespread adoption of AI in healthcare are the development of suitable datasets and the identification of best practices for machine-learning analysis.
“Physicians and scientists have long recognized the potential of AI to help understand and treat disease, but its use in clinical and research settings remains limited. This is, in part, because AI tools cannot always be easily or appropriately applied to new datasets that were not organized for this type of analysis. Furthermore, most AI algorithms function as ‘black boxes’—when they reach a conclusion about something, no one knows exactly how or why that decision was made,” explains an announcement by the University of California San Diego School of Medicine, where scientists will lead components of the National Institutes of Health (NIH) Common Fund’s Bridge to Artificial Intelligence (Bridge2AI) program.
Bridge2AI will fund $130 million over the next four years to accelerate the widespread use of AI in biomedical research and healthcare. The goal is to create comprehensive AI-ready datasets that will lay the groundwork for new, interpretable, and trustworthy AI technologies.
Healthcare and neurology leaders who monitor these and other AI advances will be best positioned to capitalize on new developments as they emerge.
—Caleb Williams
Related Information:
See How This AI Analyzes Breathing Patterns For Early Detection Of Parkinson’s
In-Home Wireless Device Tracks Disease Progression in Parkinson’s Patients